From resolving user queries to capturing online leads, customer service chatbots are a valuable asset for many businesses.
At their best, chatbots work to increase efficiency, provide instant resolutions to customers, and automate or streamline many processes.
However, not all chatbots are created equal—or capable of delivering high-quality automated customer service and support.
To measure success, track performance, and ensure your chatbot meets customer needs, you need to monitor the right metrics.
Enter chatbot analytics.
This crucial process helps you understand whether your chatbot is delivering the desired results and how to improve it further.
But what exactly are the analytics that matter?
That’s the key question we’ll be answering in this article. We’ll cover...
- What is chatbot analytics?
- 12 key metrics for tracking chatbot success
- How to measure chatbot performance using chatbot analytics tools
TL;DR: 12 important chatbot analytics metrics
- Engagement Rate: The percentage of users actively interacting with your chatbot after encountering it.
- Retention Rate: The percentage of users who return to use your chatbot after their initial interaction, indicating ongoing user engagement and satisfaction.
- Average Handling Time (AHT): The average time taken by the chatbot to resolve a user query or complete a task, reflecting efficiency.
- Average Response Time: The time it takes for your chatbot to reply to user messages, impacting user experience and satisfaction.
- Resolution Rate: The percentage of issues or queries that the chatbot resolves without human intervention, reflecting effectiveness.
- Containment Rate: The percentage of interactions fully handled by the chatbot without being escalated to human agents or other business systems.
- Customer Satisfaction (CSAT): Direct feedback from users about their experience with the chatbot, usually collected via surveys or rating systems.
- Fallback Rate: The rate at which your chatbot fails to understand or respond to queries, highlighting areas for improvement.
- Conversion Rate: The percentage of users who complete a desired action (e.g., making a purchase or signing up for a service) through the chatbot.
- Lead Capture Rate: The number of leads generated by the chatbot, reflecting its effectiveness in gathering user information or expressions of interest.
- Abandonment Rate: The percentage of chatbot interactions that users leave incomplete, often due to poor user experience.
- Cost Savings: The financial impact of using a chatbot, calculated by the reduction in customer service costs and other operational expenses.
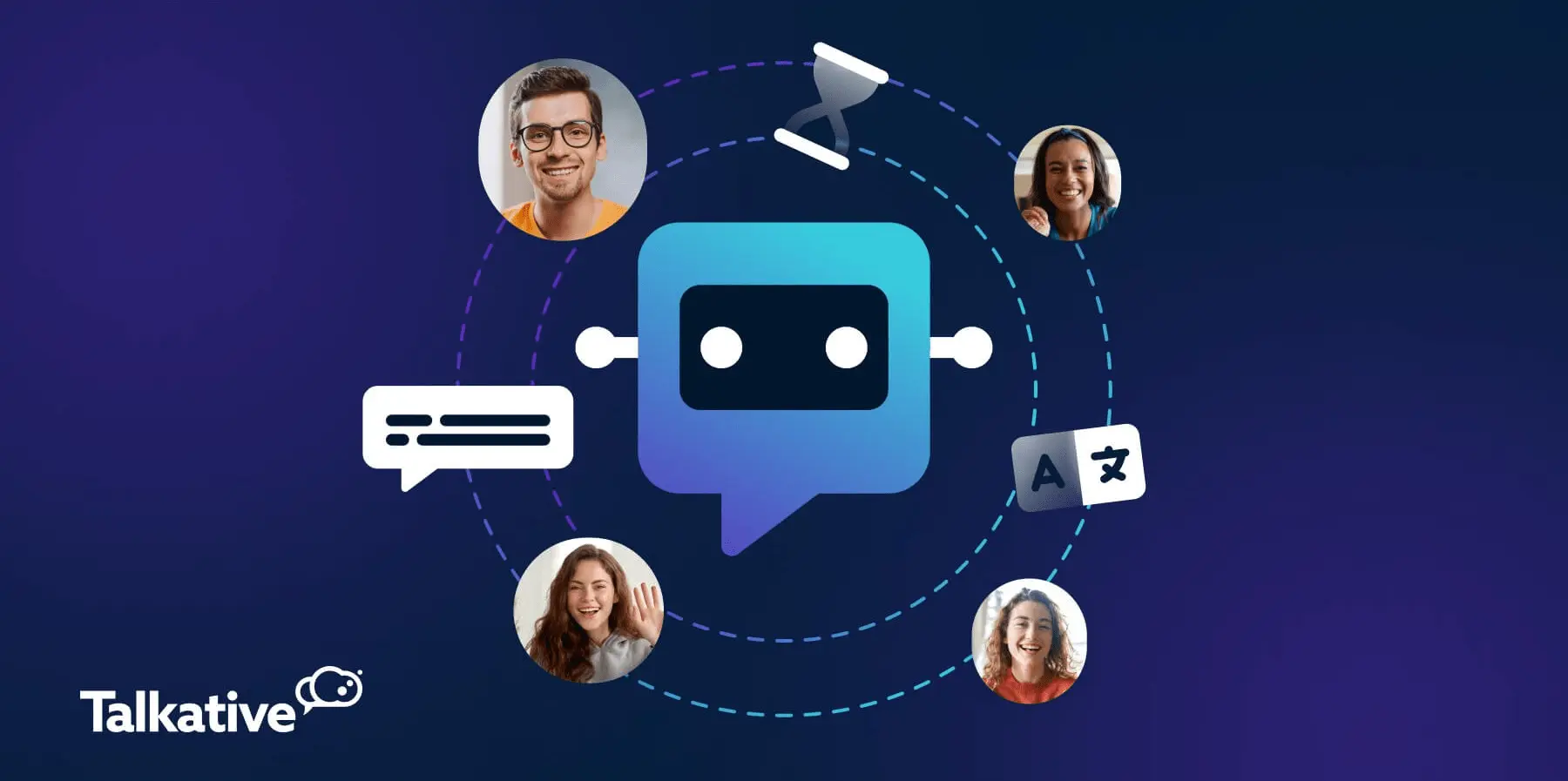
What is chatbot analytics?
Chatbot analytics is the process of gathering and analysing data from user interactions to measure performance and success.
This process is an important part of chatbot management because it provides valuable insights into how users interact with your chatbot and whether it’s meeting its intended goals.
By tracking key performance indicators (KPIs) and specific bot metrics, you can gain a deeper understanding of your chatbot solution's effectiveness and user experience.
This understanding not only highlights current achievements but also uncovers areas that require enhancement.
Below are three key ways conversational bot analytics can benefit businesses.

Monitor performance & measure success
Chatbot analytics help you assess how well your bot is adhering to its intended purpose, key goals, and objectives.
This allows you to track success in key areas such as engagement, customer satisfaction, resolution rates, lead generation, and more.
By measuring success, you can also demonstrate the tangible ROI and business value of your chatbot solution.
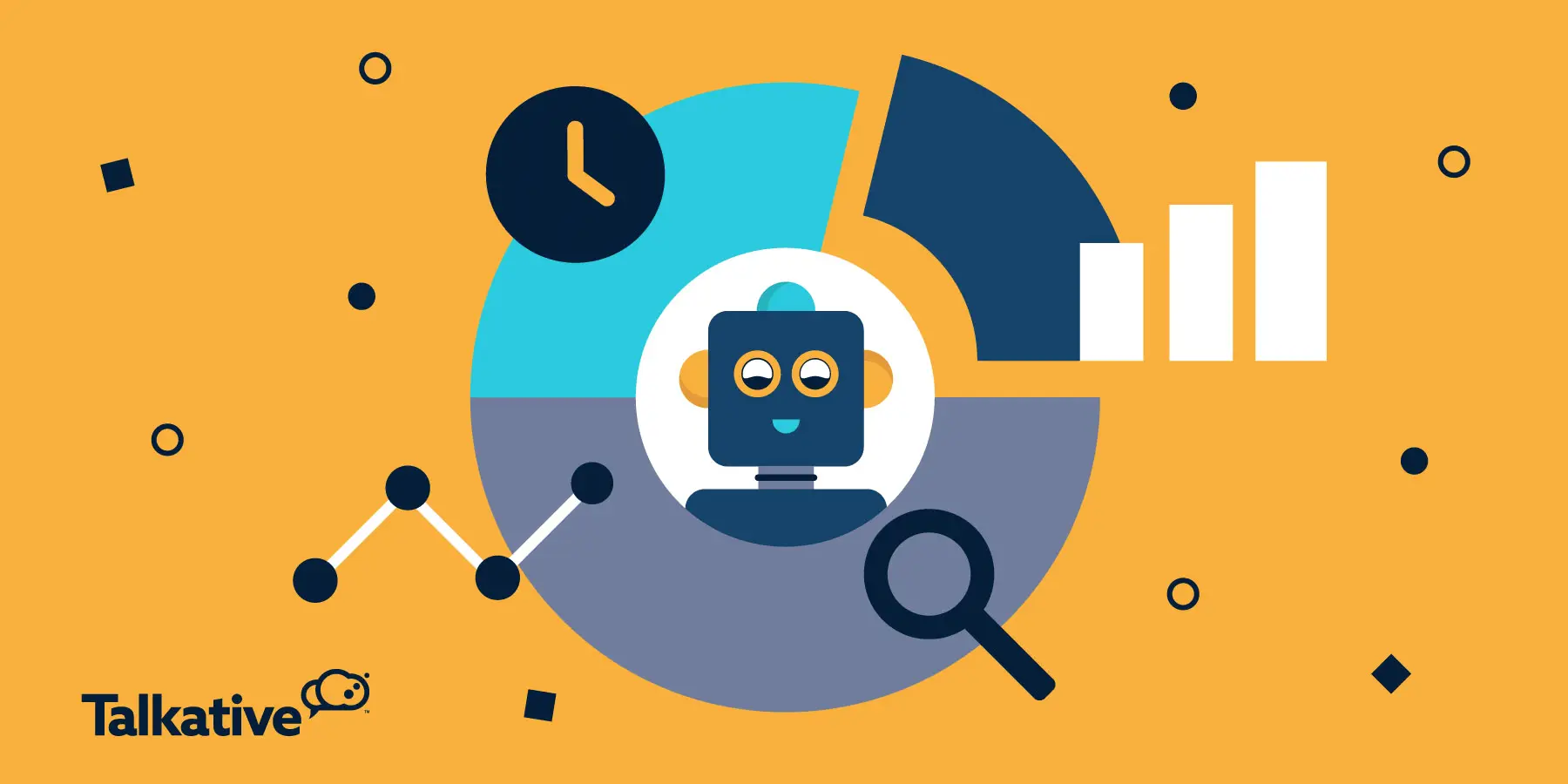
Gain insights into user engagement & experience
Chatbot analytics give you a deep understanding of how customers interact with your chatbot.
Engagement bot metrics (e.g. engagement rate, average chat duration, number of interactions, etc.) track how much and how deeply users interact with your bot.
By using analytics to segment your audience, you can also gain insights into how different groups of users engage with your bot.
This process helps you identify patterns, diverse user needs, and the ways in which engagement varies across your customer base.
It also helps you understand your chatbot’s performance and impact across different demographics, platforms, or customer journeys.
Once you’ve gathered these insights, you can target specific improvement projects to better cater to your entire audience.

Optimise chatbot performance with conversational analytics
No matter how well-designed, every chatbot encounters limitations or gaps in knowledge that impact performance.
Analytics are essential for identifying these issues and pinpointing exactly where and how your chatbot falls short.
By empowering you to measure performance and analyse user behaviour, analytics lay the foundation for continuous improvement as your chatbot evolves.
This process ensures that your bot remains aligned with changing business goals and rising customer expectations.
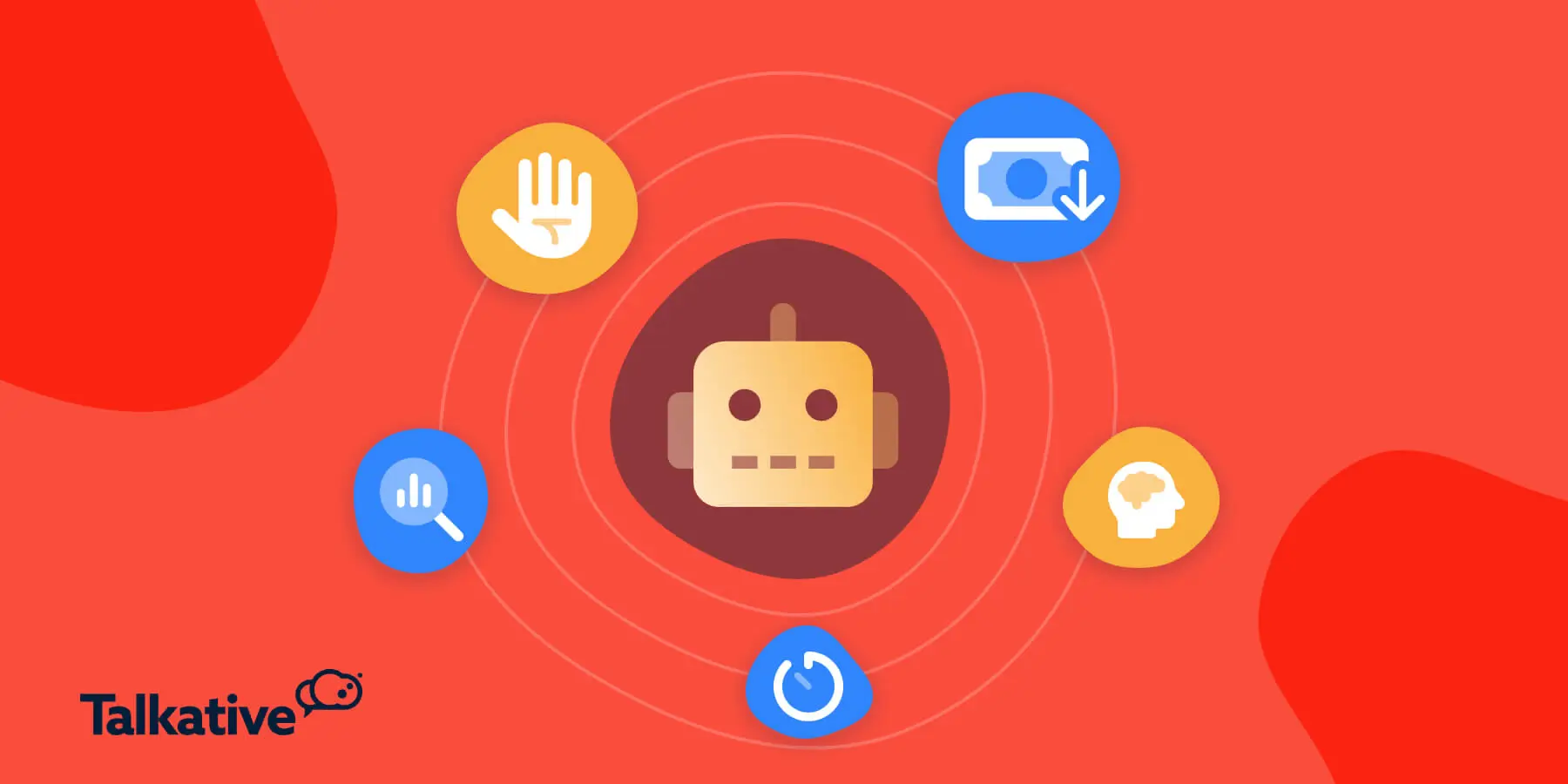
The 12 most important performance metrics for chatbots
Now that we’ve covered why analytics are important, let’s dive into the 12 most important chatbot metrics you need to track success.
1. Engagement Rate
Engagement rate measures the percentage of users who interact with your bot after encountering it.
This metric indicates how effectively your chatbot captures user interest and encourages active participation.
A high engagement rate suggests that users find your chatbot relevant and compelling.
A low engagement rate, on the other hand, might indicate poor placement, an unappealing user experience, or a misalignment between user needs and the chatbot’s offerings.
Understanding engagement rate allows you to assess the initial impact of your chatbot and make adjustments to improve its visibility and relevance, ensuring it becomes a valuable touchpoint in the customer journey.
You can calculate engagement rate using the following formula:
Engagement Rate % = (Number of Users Interacting with the Chatbot / Total Number of Users encountering the Chatbot) x 100
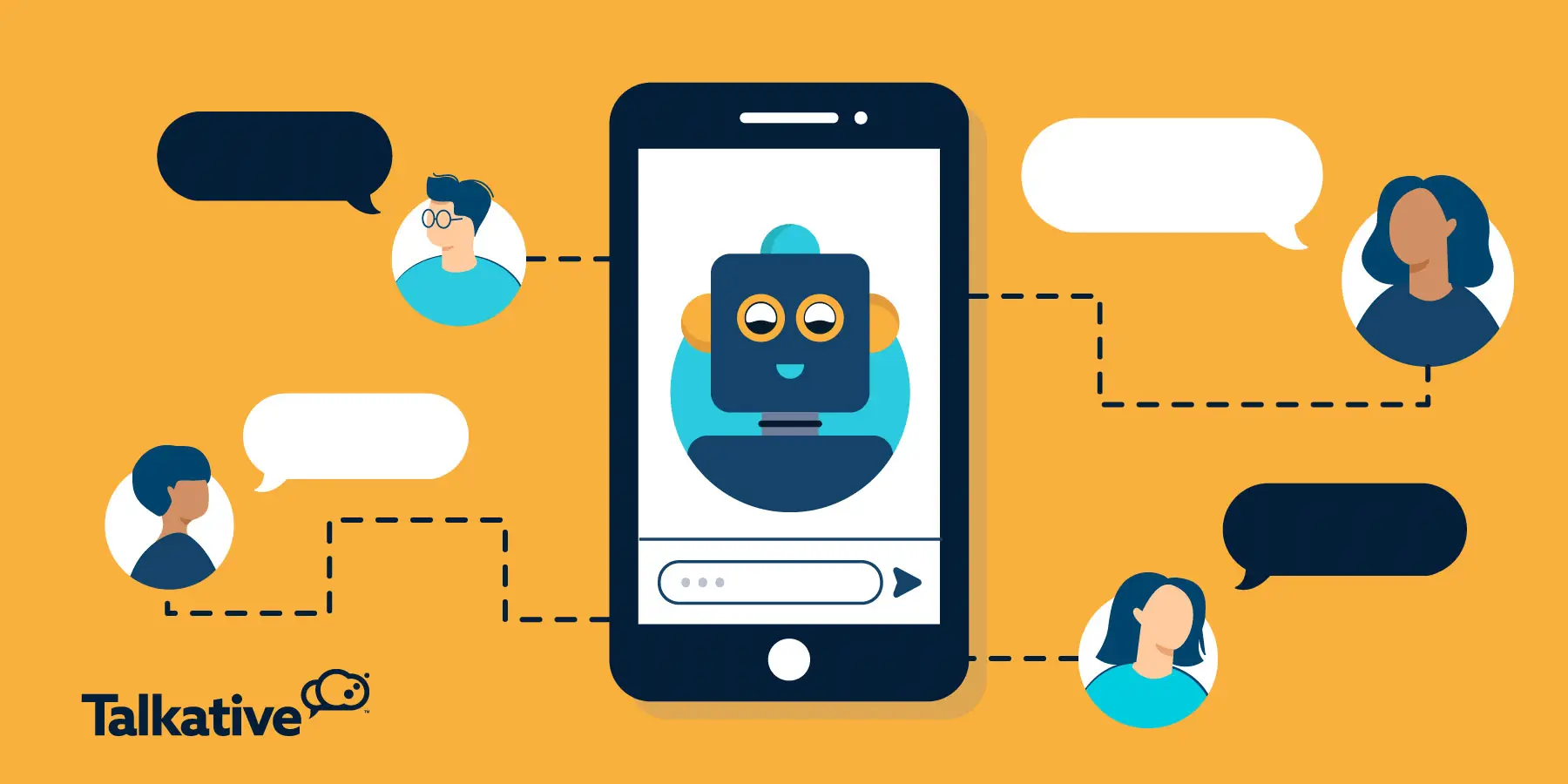
2. Retention Rate
Retention rate measures the percentage of users who return to your chatbot after their initial interaction.
Unlike engagement rate, which focuses on initial interactions, retention rate provides insight into the chatbot’s ongoing value and its ability to build lasting relationships with users.
A high retention rate indicates that users find long-term value in your chatbot, fostering customer loyalty and brand trust.
A lower retention rate may signal issues such as a lack of functionality, limited content, or unresolved user needs during initial interactions.
By tracking this metric, businesses can refine their chatbot strategy to enhance user experience, ensuring the bot not only attracts but also retains a loyal user base.
Retention rate can be calculated using the following formula:
Retention Rate % = (Number of Returning Users / Total Number of Initial Users) x 100
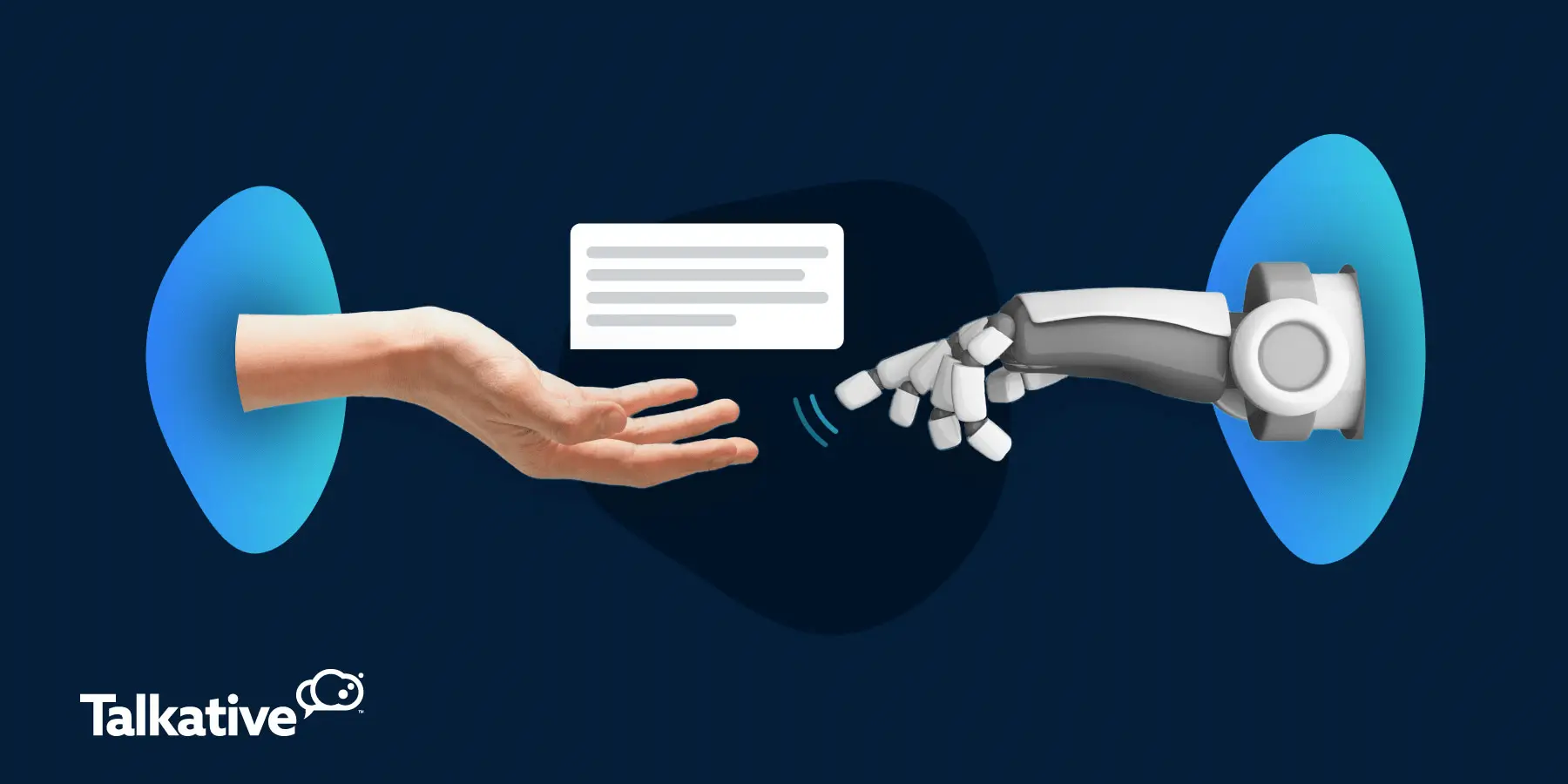
3. Average Handling Time (AHT)
Average Handling Time (AHT) measures the average duration it takes for your chatbot to fully resolve a user query or complete a task.
This metric is crucial because it reflects the efficiency of your chatbot.
A lower AHT typically indicates that the chatbot is providing concise and accurate responses, leading to a smoother customer experience and higher satisfaction.
A higher AHT might highlight issues in the conversation flow, such as repetitive prompts or insufficient information.
Monitoring AHT enables you to refine your chatbot’s performance by streamlining interaction, optimising conversation flows, and minimising delays.
The following formula can be used to calculate AHT:
Average Handling Time = Total Time Spent on Resolved Interactions / Number of Resolved Interactions
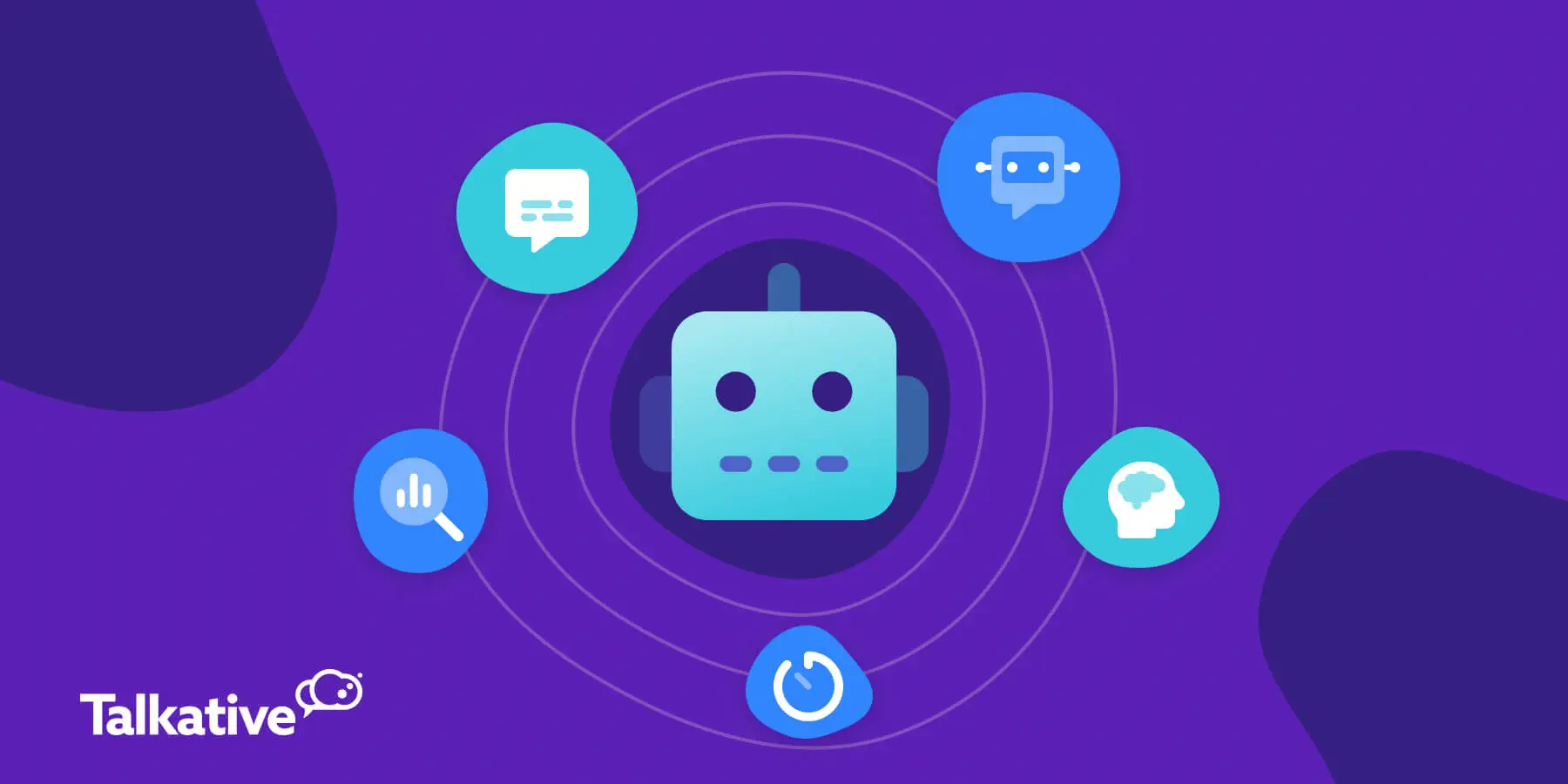
4. Average Response Time
Average response time measures how long it takes for your chatbot to reply to user messages.
Like AHT, average response time is also a key measure of efficiency as well as user experience.
A fast response time is important because it keeps users engaged, builds trust, and prevents user frustration.
A delayed response time might indicate problems with the conversation flow, an overload of user queries, or technical issues in the system.
Monitoring this metric is important because it allows you to identify and address delays in the conversation flow.
This, in turn, ensures a smoother, more human-like AI experience that meets customer expectations and boosts user satisfaction.
The following formula can be used to calculate average response time:
Average response Time = Total Time Taken to Respond to All User Messages / Total Number of User Messages
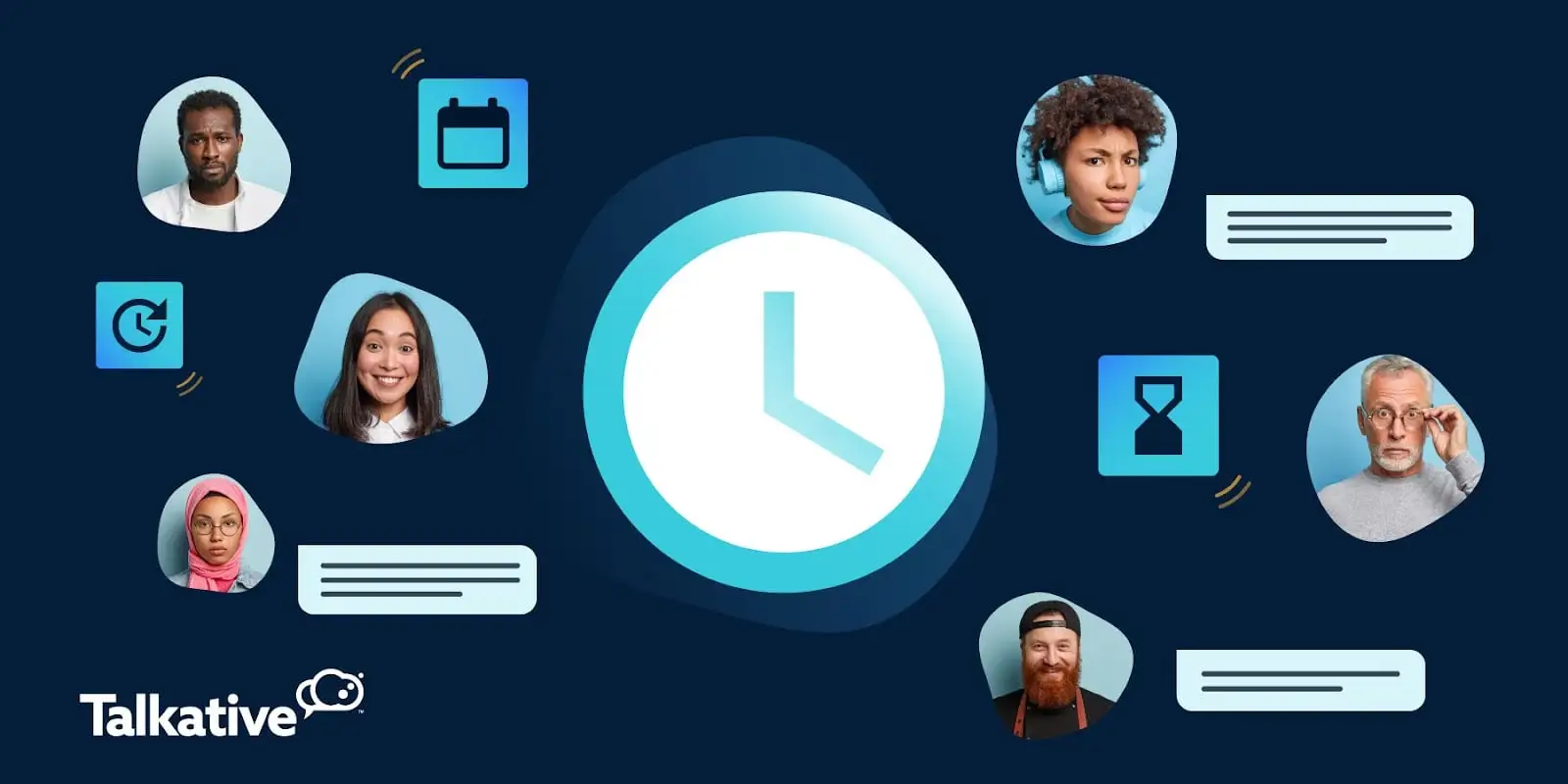
5. Resolution Rate
Resolution rate measures the percentage of user queries or issues that are fully resolved by your chatbot without human assistance or follow-up.
This metric provides insight into how effective your chatbot is at delivering successful outcomes.
A high resolution rate indicates a well-designed and informative chatbot that effectively leverages a comprehensive knowledge base.
A low resolution rate highlights gaps in your chatbot’s capabilities or content.
By tracking resolution rate, you can identify areas where your chatbot needs improvement.
In turn, you’ll be able to optimise the bot’s performance to better empower users with self-service and seamless automated support.
Thanks to advancements in technologies like conversational AI and generative AI, today’s chatbots can excel in this area.
For example, Talkative customer Healthspan has managed to reach a 90% chatbot resolution rate for product queries.
The following formula can be used to calculate resolution rate:
Resolution Rate % = (Number of Issues Fully Resolved by the Chatbot / Total Number of User Interactions) x 100
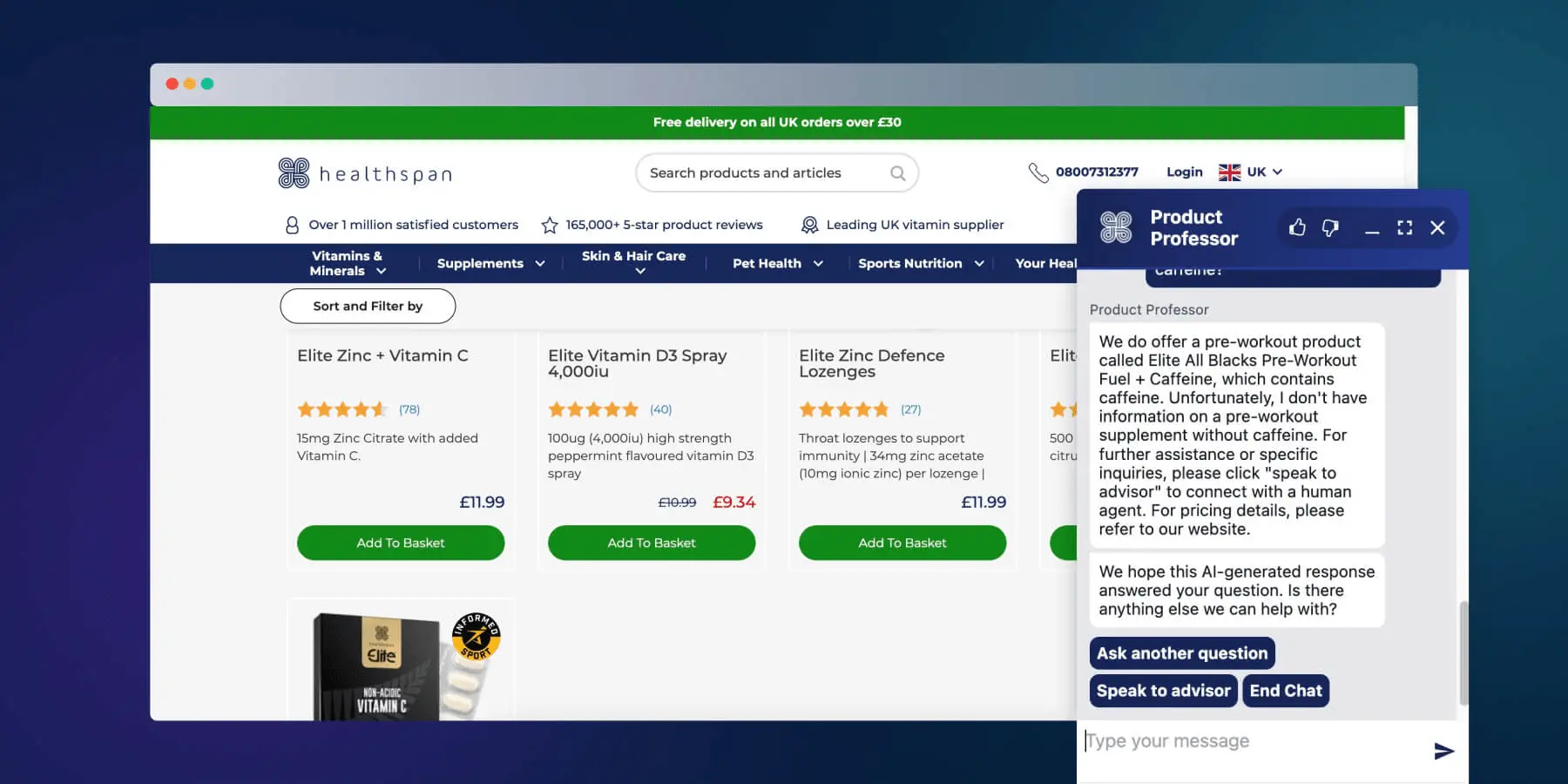
6. Containment Rate
Chatbot containment rate measures the percentage of user interactions that are handled exclusively by the chatbot without human assistance or intervention from other business systems.
This metric is similar to resolution rate in that it reflects how effective your chatbot is at managing customer interactions.
High containment rates indicate that your chatbot is capable of addressing a wide range of interactions independently.
Lower rates may highlight gaps in your chatbot’s knowledge base or functionality.
Measuring containment rate provides an understanding of your chatbot’s ability to manage customer inquiries.
It also helps you identify areas for improvement, such as expanding the bot’s capabilities or integrating it more seamlessly with other business systems.
The following formula can be used to calculate containment rate:
Containment Rate % = (Number of Interactions Fully Handled by the Chatbot / Total Number of User Interactions) x 100
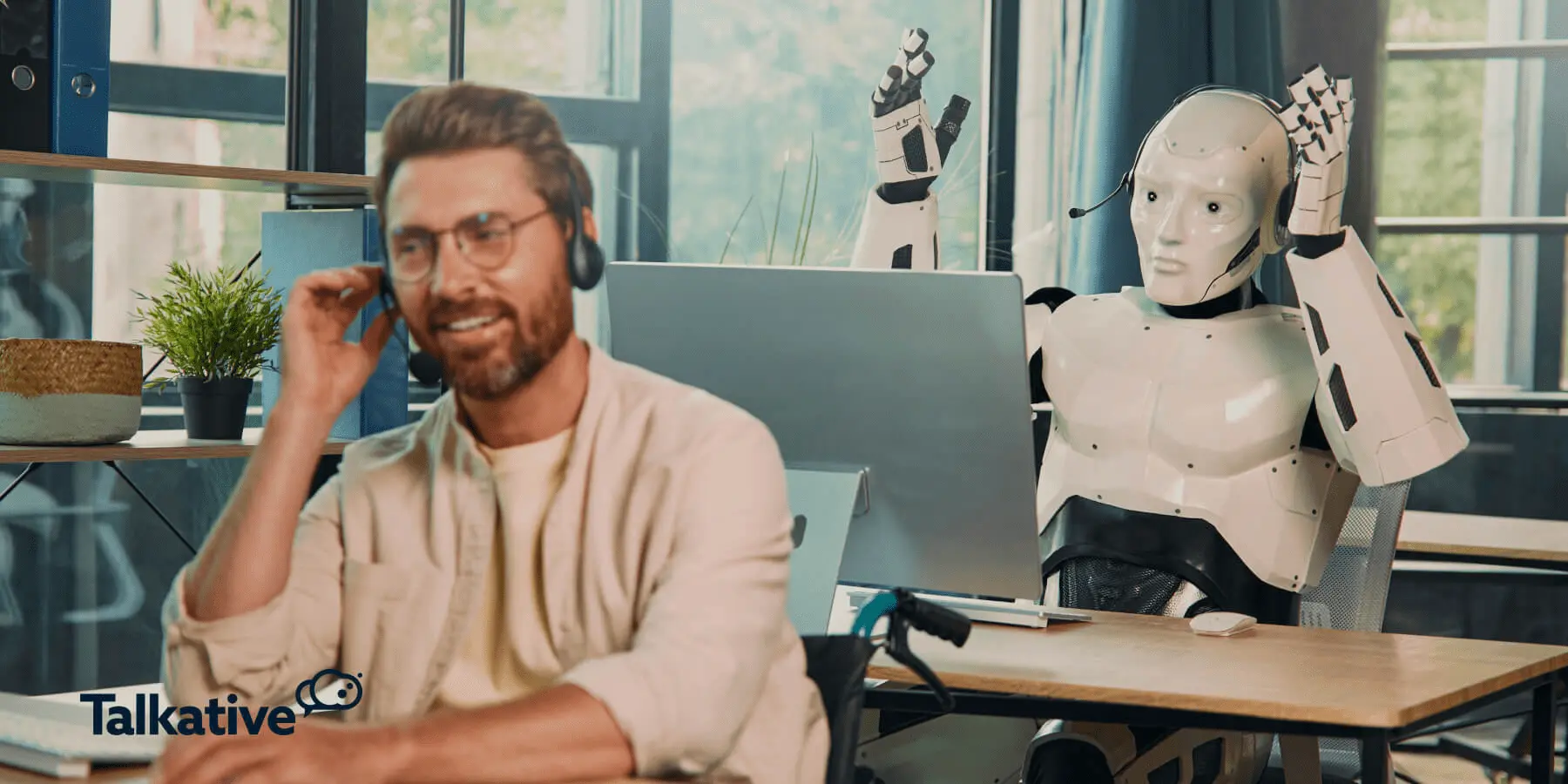
7. Customer Satisfaction (CSAT) Score
Customer satisfaction score (CSAT) is a direct measure of how pleased users are with their interaction with your chatbot.
Typically collected through post-interaction surveys or rating systems, CSAT provides immediate feedback on user experience.
A high CSAT score indicates that the chatbot is meeting or exceeding user expectations in terms of functionality, speed, and overall experience.
Low satisfaction scores highlight pain points, such as unresolved issues, slow response times, or a poor conversational experience.
By tracking CSAT, you can gain direct feedback from users, allowing you to make targeted improvements.
This helps ensure that your chatbot not only meets user needs but also fosters positive brand perception and customer loyalty.
The formula for calculating Customer Satisfaction (CSAT) is:
CSAT % = (Number of Satisfied Customers / Total Number of Feedback Responses) × 100

8. Fallback Rate
Fallback rate measures the percentage of instances where your chatbot fails to understand or respond to a user query.
This metric is important because it highlights the limitations in your chatbot’s understanding or knowledge base.
A high fallback rate indicates that users frequently encounter queries or inputs that the chatbot can’t handle.
This typically stems from gaps in training data, poor conversation design, or unexpected user inputs.
A low fallback rate, on the other hand, signifies a well-optimised chatbot capable of handling a wide range of user inquiries.
By monitoring fallback rate, you can identify and address the gaps in your chatbot’s capabilities.
Fallback rate can be calculated using this formula:
Fallback Rate % = (Number of Fallback Responses / Total Number of User Messages) x 100

9. Conversion Rate
Conversion rate tracks the percentage of chatbot users who complete a desired action through the chatbot.
This desired action can be any key goal you set, such as making a purchase, scheduling an appointment, signing up for a newsletter, etc.
Understanding conversion rate is important because it directly links your chatbot’s performance to business outcomes.
A high conversion rate indicates that the chatbot is effectively guiding users through the customer journey and persuading them to take action.
A low conversion rate, on the other hand, suggests a poor conversation flow, user experience, or misalignment between your chatbot’s offerings and user needs.
By monitoring conversion rate, you can discover how well your chatbot is driving user actions and make targeted improvements to boost its impact on your business success.
The following formula can be used to calculate conversion rate:
Conversion Rate % = (Number of Users Who Complete the Desired Action / Total Number of Users Interacting with the Chatbot) x 100

10. Lead Capture Rate
Lead capture rate measures the effectiveness of your chatbot in gathering user information for lead generation.
This metric is particularly important for marketing and sales chatbots, where the goal is to secure potential customer data for follow-up.
A high lead capture rate indicates that your chatbot is successfully engaging users and capturing valuable information, such as contact details or specific inquiries.
A low lead rate might suggest a need for improvement in the conversational flow, incentives, or the clarity of your chatbot’s prompts.
By tracking lead generation, you can optimise your chatbot’s ability to gather information, refine its scripts, and enhance its alignment with user intent—ultimately boosting your sales pipeline.
Lead capture rate can be calculated using the following formula:
Lead Rate % = (Number of Leads Captured by the Chatbot / Total Number of User Interactions) x 100

11. Abandonment Rate
Abandonment rate measures the percentage of chatbot interactions that are left incomplete by users.
This metric highlights instances where users disengage from or terminate a chat before reaching a resolution or completing a task.
A high abandonment rate indicates user frustration or dissatisfaction, often stemming from a poor conversation flow, lengthy interactions, or a chatbot’s inability to address queries effectively.
A low abandonment rate suggests that your chatbot is successful in maintaining user engagement and delivering satisfactory outcomes.
By monitoring abandonment rate, you can identify and address pain points in the user experience.
This might include improving response times, enhancing the bot’s knowledge base, or refining conversation flows to reduce friction and increase user satisfaction.
Abandonment rate can be calculated using the following formula:
Abandonment Rate % = (Number of Incomplete Interactions / Total Number of User Interactions) x 100

12. Cost Savings
Cost savings is a business-impact metric that measures the financial benefits gained from deploying a chatbot.
This metric quantifies the reduction in operational expenses, particularly in customer service, as a result of automated interactions.
Chatbots can significantly decrease the need for human support, leading to savings in labour costs, training, and resources.
By calculating cost savings, businesses can assess the direct impact of a chatbot on their bottom line and showcase chatbot ROI.
The formula for calculating cost savings from a chatbot is generally as follows:
Cost Savings = (Number of Interactions Handled by the Chatbot x Cost Per Interaction with a Human Agent) - Deployment and Maintenance Costs of the Chatbot

How to track performance: Chatbot analytics tools
Understanding and leveraging chatbot analytics is crucial for maximising the impact of your bot.
This process is made easy and efficient with the right tools and AI analytics platform.
Many of these tools/platforms offer comprehensive reporting, real-time insights, and an intuitive chatbot analytics dashboard.
At Talkative, we provide our own advanced, in-depth reporting and analytics.
Our dashboards, reports, and chatbot analytics platform include the following key features...
- Real-time chatbot analytics and reporting, enabling you to monitor live user interactions, track key performance indicators, and gain actionable insights instantly as conversations happen.
- Interactive dashboard filters, allowing you to refine chatbot data analytics results by date and time range, engagement type (e.g., proactive vs. manual interaction), user location, browser, OS, device, and returning users.
- Knowledge base reporting that analyses gaps in your chatbot’s knowledge base to refine training and make targeted updates that will improve the content and performance of your knowledge base.
- An internal-facing AI Analytics Assistant bot that you can ask for specific insights, tailored reports, and instant data summaries.

The takeaway
Chatbot analytics is a crucial best practice for measuring success, understanding user interactions, and improving both performance and the customer experience.
By tracking the 12 key chatbot metrics outlined above, you can gain a comprehensive understanding of how your chatbot contributes to business goals and customer satisfaction.
But if you want to get the most out of your chatbot, you also need it to be powered by the right technology.
That’s where Talkative comes in.
With our scalable and flexible AI chatbot solution, you can...
- Leverage an AI-powered or rule-based solution (or a combination of the two - an AI chatbot with rule-based fall-back for maximum efficiency)
- Choose between multiple LLMs, including LLAMA, Claude, Google Gemini, and OpenAI GPT models.
- Gain access to a huge range of AI reports and advanced analytics features
- Create multiple AI knowledge bases that make your chatbot an expert in your brand, products, and services
- Meet and serve customers across your website, app, and social messaging platforms
- Seamlessly escalate to human agents when needed
- Provide multilingual customer service without any additional downloads or plugins
In addition to AI chatbots, we offer a suite of customer contact channels and capabilities - including live chat, web calling, video chat, cobrowse, messaging, and more.
Want to learn more?
Book a demo with Talkative today, and check out our interactive product tour.